In This Post
- 1 Table of Contents
- 2 What is Artificial Intelligence
- 3 “Can machines think?”
- 4 “How does AI accelerate fundamental research?”
- 5 AI Research Use Cases
- 5.1 Automated Data
- 5.2 Optimize Equipment
- 5.3 Healthcare
- 5.4 Computer Science
- 5.5 Large-scale machine learning
- 5.6 Deep learning
- 5.7 Reinforcement learning
- 5.8 Robotics
- 5.9 Computer vision
- 5.10 Natural Language Processing
- 5.11 Collaborative systems
- 5.12 Crowdsourcing and human computation
- 5.13 Algorithmic game theory and computational social choice
- 5.14 Internet of Things (IoT)
- 5.15 Neuromorphic Computing
- 6 The math behind Machine Learning Algorithms
- 7 Modern Challenges with Machine Learning and Artificial Intelligence take the front seat in Scientific Research
- 8 Principles to Build Trust in Artificial Intelligence Domain
- 9 Can humans make Artificial Intelligence approach Principled
- 10 Top Artificial Intelligence Leaders and Researchers
Table of Contents
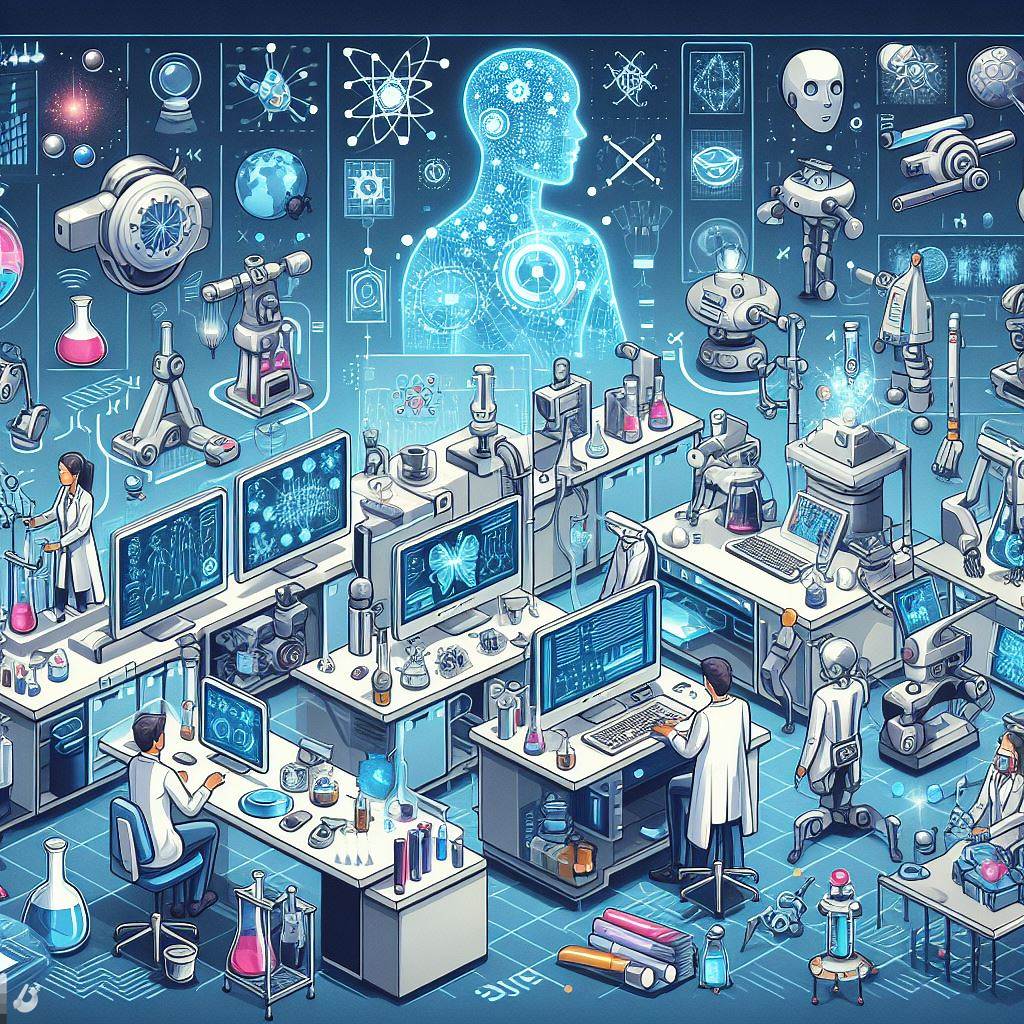
What is Artificial Intelligence
According to Wikipedia: Artificial intelligence (AI) is the intelligence of machines or software, as opposed to the intelligence of humans or animals. It may also refer to the corresponding field of study, which develops and studies intelligent machines, or to the intelligent machines themselves.
“Can machines think?”
The question of whether machines can think has been a topic of discussion for decades. One of the most famous attempts to answer this question is the Turing test, which was proposed by Alan Turing in 1950 1. The Turing test is a test of a machine’s ability to exhibit intelligent behavior equivalent to, or indistinguishable from, that of a human. The test involves a human evaluator judging natural language conversations between a human and a machine designed to generate human-like responses. If the evaluator cannot reliably tell the machine from the human, the machine would be said to have passed the test 1.
However, the Turing test has been criticized for being too narrow a definition of intelligence and for not taking into account other aspects of intelligence such as creativity, emotions, and consciousness 2. Some experts argue that machines can never truly think because they lack the subjective experience of consciousness that humans possess 3. Others believe that machines can think, but that their thinking is fundamentally different from human thinking .
In conclusion, while machines are capable of mimicking human thoughts and behaviors to a certain extent, the question of whether they can truly think like humans remains a matter of debate.
“How does AI accelerate fundamental research?”
New research and applications are emerging rapidly with the support by AI infrastructure, including data storage, computing power, AI algorithms, and frameworks.
The field of artificial intelligence is rapidly evolving, with new research and applications emerging every day. AI infrastructure, including data storage, computing power, AI algorithms, and frameworks, is advancing at an unprecedented pace, enabling machines to perform increasingly complex tasks. For example, by 2040, the convergence of technologies such as AI, high-speed telecommunications, and biotechnology will enable rapid breakthroughs and user-customized applications that are far more than the sum of their parts 1. Generative design AI is expected to automate 60% of the design effort for new websites and mobile apps by 2026, and over 100 million humans will engage robocolleagues to contribute to their work 2. The potential of AI is vast, and its impact on society is only just beginning to be realized.
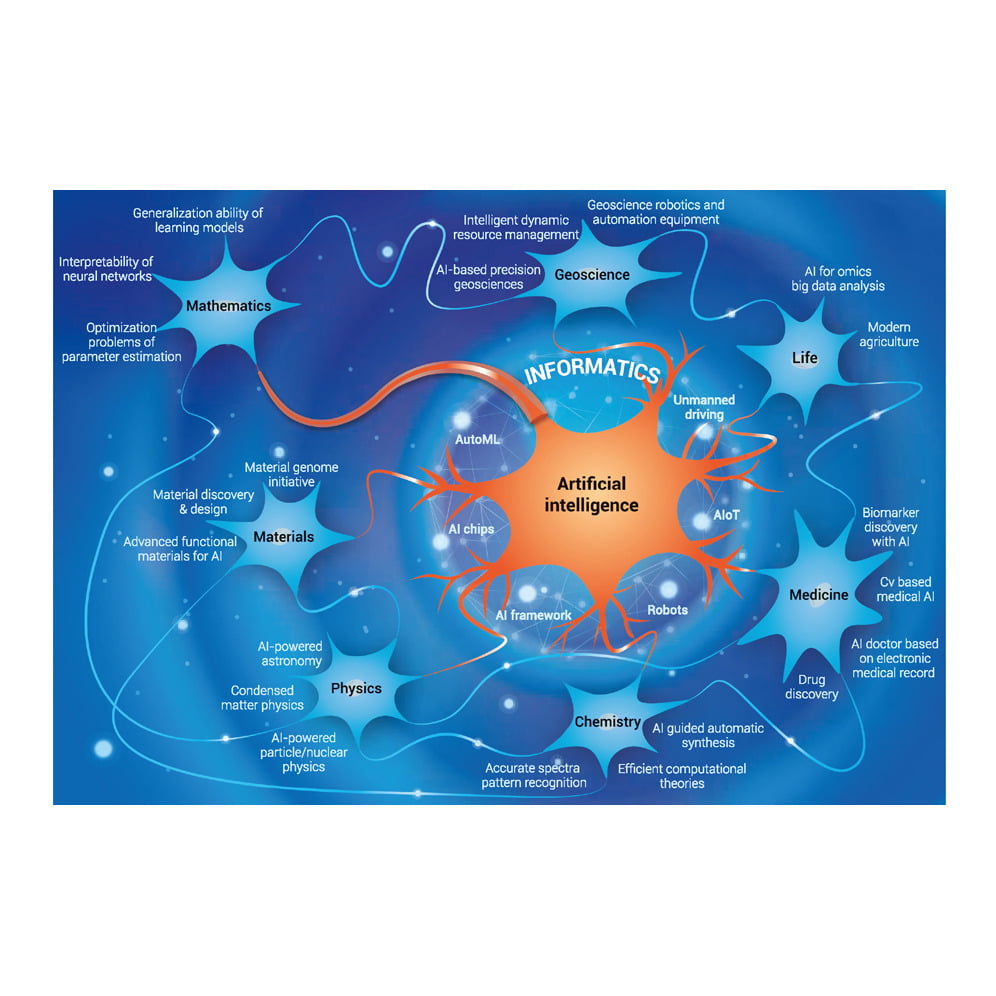
AI Research Use Cases
A major advancement in artificial intelligence research recently came with a machine learning algorithm capable of inventing radical new proteins that can fight disease. Also, AI researchers are now developing algorithms that can search for scientific research papers and extract information from them to automatically correct scientific papers. Let’s take a look at some more uses cases of AI in research.
Automated Data
Artificial intelligence is also used to optimize resources in research laboratories, automate the acquisition of data and facilitate the synthesis and analysis of complex datasets. For example, AI has recently been used to help manage the activities in large-scale, long-term studies by providing real-time guidance. An AI system may be able to monitor the health of each participant in a study and alert a scientist if a participant’s status changes.
Optimize Equipment
AI is also being used to optimize laboratory techniques and equipment. AI-driven robots can automatically perform several tasks that were previously only carried out by humans, such as organizing and storing scientific equipment, preparing samples for analysis, and carrying out routine diagnostic tests. In addition, automated systems are also able to carry out tasks that are too dangerous or difficult for scientists or technicians to complete themselves. AI and robotics are also being used in the design of experiments—helping researchers determine which parameters should be changed, how the experiment should be designed, and what measurements should be made.
Healthcare
Many believe AI will soon be used to identify new drugs and drug combinations, diagnose diseases from medical images, and assist in surgeries. AI was used to predict an enzyme better than any other prediction before. A technique called deep learning was used. The system was able to predict the three-dimensional structure of an enzyme. The most important thing is that the 3D structure was more complex than those the algorithm was previously trained to deal with. Artificial intelligence has also been successfully used in cancer research to create better ways to detect, diagnose, and treat cancer patients.
Researchers reported that they used machine vision to analyze human behavior and physical characteristics in videos of people with autism and Asperger’s Syndrome. They used AI algorithms based on deep learning with a dataset of 1,200 videos featuring 12-megapixel cameras, just like the ones on iPhone 13, and individuals making facial expressions or engaged in social interactions, such as smiling or nodding. The analysis revealed ten distinct facial states of autism, while deep neural networks also accurately projected the severity of symptoms.
Computer Science
Researchers use AI-based algorithms to search databases of molecules and find effective molecules with desired properties. Such an algorithm may be able to search databases of millions of molecules in a fraction of the time it would take an expert scientist.
Computer scientists also created a system that can be used to generate new educational games based on existing video games. The researchers used AI to develop new algorithms for recombining existing game elements into new types of games. They used machine learning to create the system, which uses a personalized learning algorithm to select elements from a large amount of video game content and then recombines them in an unpredictable way. The researchers suggest that this technique could be useful for exploring different genres of video games or creating new genres based on already existing ones.
Large-scale machine learning
Many of the basic problems in machine learning (such as supervised and unsupervised learning) are well-understood. A major focus of current efforts is to scale existing algorithms to work with extremely large data sets. For example, whereas traditional methods could afford to make several passes over the data set, modern ones are designed to make only a single pass; in some cases, only sublinear methods (those that only look at a fraction of the data) can be admitted.
Deep learning
The ability to successfully train convolutional neural networks has most benefited the field of computer vision, with applications such as object recognition, video labeling, activity recognition, and several variants thereof. Deep learning is also making significant inroads into other areas of perception, such as audio, speech, and natural language processing.
Reinforcement learning
Traditional machine learning has primarily focused on pattern mining, whereas reinforcement learning emphasizes decision-making and is a technology that will help AI advance more deeply into the realm of learning about and executing actions in the real world. Reinforcement learning has existed for several decades as a framework for experience-driven sequential decision-making, but the methods have not found great success in practice, mainly owing to issues of representation and scaling. However, the advent of deep learning has provided reinforcement learning with a “shot in the arm.” The recent success of AlphaGo, a computer program developed by Google Deepmind that beat the human Go champion in a five-game match, was due in large part to reinforcement learning. AlphaGo was trained by initializing an automated agent with a human expert database, but was subsequently refined by playing a large number of games against itself and applying reinforcement learning.
Robotics
Robotic navigation, at least in static environments, is largely solved. Current efforts consider how to train a robot to interact with the world around it in generalizable and predictable ways. A natural requirement that arises in interactive environments is manipulation, another topic of current interest. The deep learning revolution is only beginning to influence robotics, in large part because it is far more difficult to acquire the large labeled data sets that have driven other learning-based areas of AI. Reinforcement learning (see above), which obviates the requirement of labeled data, may help bridge this gap but requires systems to be able to safely explore a policy space without committing errors that harm the system itself or others. Advances in reliable machine perception, including computer vision, force, and tactile perception, much of which will be driven by machine learning, will continue to be key enablers to advancing the capabilities of robotics.
Computer vision
Computer vision is currently the most prominent form of machine perception. It has been the sub-area of AI most transformed by the rise of deep learning. Until just a few years ago, support vector machines were the method of choice for most visual classification tasks. But the confluence of large-scale computing, especially on GPUs, the availability of large datasets, especially via the internet, and refinements of neural network algorithms has led to dramatic improvements in performance on benchmark tasks (e.g., classification on ImageNet[17]). For the first time, computers are able to perform some (narrowly defined) visual classification tasks better than people. Much current research is focused on automatic image and video captioning.
Natural Language Processing
Often coupled with automatic speech recognition, Natural Language Processing is another very active area of machine perception. It is quickly becoming a commodity for mainstream languages with large data sets. Google announced that 20% of current mobile queries are done by voice,[18] and recent demonstrations have proven the possibility of real-time translation. Research is now shifting towards developing refined and capable systems that are able to interact with people through dialog, not just react to stylized requests.
Collaborative systems
Research on collaborative systems investigates models and algorithms to help develop autonomous systems that can work collaboratively with other systems and with humans. This research relies on developing formal models of collaboration, and studies the capabilities needed for systems to become effective partners. There is growing interest in applications that can utilize the complementary strengths of humans and machines—for humans to help AI systems to overcome their limitations, and for agents to augment human abilities and activities.
Crowdsourcing and human computation
Crowdsourcing and human computation are research areas that aim to augment computer systems by utilizing human intelligence to solve problems that computers alone cannot solve well. This research has gained an established presence in AI over the past fifteen years. Wikipedia is a well-known example of crowdsourcing, which is a knowledge repository maintained and updated by netizens and that far exceeds traditionally-compiled information sources, such as encyclopedias and dictionaries, in scale and depth. Crowdsourcing focuses on devising innovative ways to harness human intelligence. Citizen science platforms energize volunteers to solve scientific problems, while paid crowdsourcing platforms such as Amazon Mechanical Turk provide automated access to human intelligence on demand. Work in this area has facilitated advances in other subfields of AI, including computer vision and NLP, by enabling large amounts of labeled training data and/or human interaction data to be collected in a short amount of time. Current research efforts explore ideal divisions of tasks between humans and machines based on their differing capabilities and costs.
The economic and social computing dimensions of AI, including incentive structures, are receiving increased attention. Since the early 1980s, distributed AI and multi-agent systems have been studied, gaining prominence in the late 1990s and accelerated by the internet. A natural requirement is that systems handle potentially misaligned incentives, including self-interested human participants or firms, as well as automated AI-based agents representing them. Topics receiving attention include computational mechanism design, which is an economic theory of incentive design that seeks incentive-compatible systems where inputs are truthfully reported. Computational social choice is a theory for how to aggregate rank orders on alternatives. Incentive-aligned information elicitation includes prediction markets, scoring rules, and peer prediction. Algorithmic game theory studies the equilibria of markets, network games, and parlor games such as Poker, where significant advances have been made in recent years through abstraction techniques and no-regret learning.
Internet of Things (IoT)
A lot of research is being conducted on the idea that a wide range of devices can be connected to collect and share their sensory information. These devices can include appliances, vehicles, buildings, cameras, and other things. While it’s a matter of technology and wireless networking to connect the devices, AI can process and use the resulting huge amounts of data for intelligent and useful purposes. Currently, these devices use a bewildering array of incompatible communication protocols. AI could help tame this Tower of Babel.
Neuromorphic Computing
Traditional computers follow the von Neumann model of computing, which divides the modules for input/output, instruction-processing, and memory. However, with the success of deep neural networks on a wide range of tasks, manufacturers are exploring alternative models of computing that are inspired by biological neural networks. These “neuromorphic” computers aim to improve the hardware efficiency and robustness of computing systems. Although they have not yet demonstrated significant advantages over traditional computers, they are becoming commercially viable. It is possible that they will become commonplace in the near future, even if only as additions to their von Neumann counterparts. Deep neural networks have already made a significant impact in the application landscape. When these networks can be trained and executed on dedicated neuromorphic hardware, rather than simulated on standard von Neumann architectures, a larger wave may hit.
Reference and Further Reading: AI Research Use Cases AI Research Trends
The math behind Machine Learning Algorithms
Supervised Learning
Supervised machine learning builds a model that makes predictions based on evidence in the presence of uncertainty. A supervised learning algorithm takes a known set of input data and known responses to the data (output) and trains a model to generate reasonable predictions for the response to new data. Use supervised learning if you have known data for the output you are trying to predict.
Supervised learning uses classification and regression techniques to develop machine learning models.
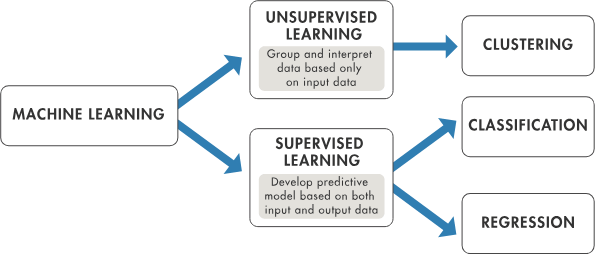
Unsupervised Learning
Unsupervised learning finds hidden patterns or intrinsic structures in data. It is used to draw inferences from datasets consisting of input data without labeled responses.
Clustering is the most common unsupervised learning technique. It is used for exploratory data analysis to find hidden patterns or groupings in data. Applications for cluster analysis include gene sequence analysis, market research, and object recognition.
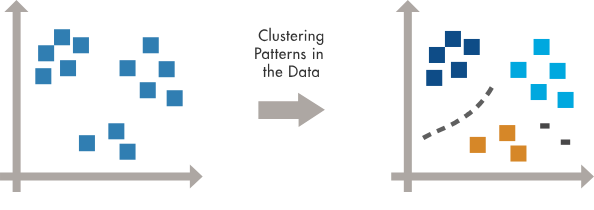
Reference and Further Reading: The math behind Machine Learning Algorithms
Modern Challenges with Machine Learning and Artificial Intelligence take the front seat in Scientific Research
Artificial-intelligence tools are transforming data-driven science — better ethical standards and more robust data curation are needed to fuel the boom and prevent a bust.
Garbage in, garbage out: mitigating risks and maximizing benefits of AI in research
Garbage in, garbage out: mitigating risks and maximizing benefits of AI in research
Artificial-intelligence tools are transforming data-driven science — better ethical standards and more robust data curation are needed to fuel the boom and prevent a bust.
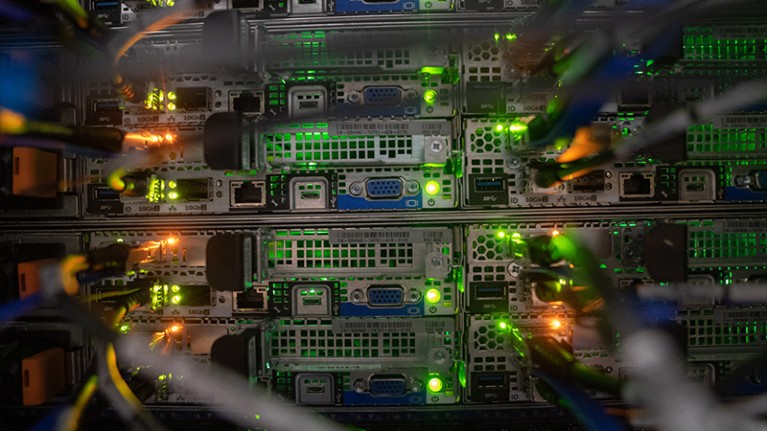
Science is producing an overwhelming amount of data that is difficult to comprehend. To make sense of this information, artificial intelligence (AI) is increasingly being utilized (see ref. 1 and Nature Rev. Phys. 4, 353; 2022). Machine-learning (ML) methods are becoming more adept at identifying patterns without explicit programming by training on vast amounts of data.
In the field of Earth, space, and environmental sciences, technologies such as sensors and satellites are providing detailed views of the planet, its life, and its history at all scales. AI tools are being applied more widely for weather forecasting2 and climate modeling3, managing energy and water4, and assessing damage during disasters to expedite aid responses and reconstruction efforts.
The rise of AI in the field is evident from the number of abstracts5 at the annual conference of the American Geophysical Union (AGU), which typically attracts over 25,000 Earth and space scientists from more than 100 countries. The number of abstracts mentioning AI or ML has increased more than tenfold between 2015 and 2022, from less than 100 to around 1,200 (that is, from 0.4% to more than 6%; see ‘Growing AI use in Earth and space science’)6.
To prevent AI technology from damaging science and public trust, an independent scientific body should be established to test and certify generative artificial intelligence.
Governments are beginning to regulate AI technologies, but comprehensive and effective legislation is years away (see Nature 620, 260–263; 2023). The draft European Union AI Act (now in the final stages of negotiation) demands transparency, such as disclosing that content is AI-generated and publishing summaries of copyrighted data used for training AI systems. The administration of US President Joe Biden aims for self-regulation. In July, it announced that it had obtained voluntary commitments from seven leading tech companies “to manage the risks posed by AI systems”.
Reference and Further Reading: Modern Challenges
Principles to Build Trust in Artificial Intelligence Domain
1. Transparency. Clearly document and report participants, data sets, models, bias and uncertainties.
2. Intentionality. Ensure that the AI model and its implementations are explained, replicable and reusable.
3. Risk. Consider and manage the possible risks and biases that data sets and algorithms are susceptible to, and how they might affect the outcomes or have unintended consequences.
4. Participatory methods. Ensure inclusive research design, engage with communities at risk and include domain expertise.
5. Outreach, training, and leading practices. Provide for all roles and career stages.
6. Sustained effort. Implement, review and advance these guidelines.
Can humans make Artificial Intelligence approach Principled
In the field of AI safety, researchers are exploring different approaches to ensure that AI systems learn human values and act in accordance with them. One such approach is called value alignment, which was pioneered by AI scientist Stuart Russell at the University of California, Berkeley. The idea behind value alignment is to train AI systems to learn human values and act in accordance with them. One of the advantages of this approach is that it could be developed now and applied to future systems before they present catastrophic hazards1.
However, some critics argue that value alignment focuses too narrowly on human values when there are many other requirements for making AI safe. For example, a foundation of verified, factual knowledge is essential for AI systems to make good decisions, just as it is for humans. According to Oren Etzioni, a researcher at the Allen Institute for AI, “The issue is not that AI’s got the wrong values. The truth is that our actual choices are functions of both our values and our knowledge” 1.
With these criticisms in mind, other researchers are working to develop a more general theory of AI alignment that works to ensure the safety of future systems without focusing as narrowly on human values.
Top Artificial Intelligence Leaders and Researchers
- Andrew Ng
- Fei-Fei Li
- Andrej Karpathy
- Demis Hassabis
- Ian Goodfellow
- Yann LeCun
- Jeremy Howard
- Ruslan Salakhutdinov
- Geoffrey Hinton
- Alex Smola
- Rana el Kaliouby
- Daphne Koller
References and Further Reading: Leaders and Researchers